CLEVELAND — Despite advances in treatment, acute myeloid leukemia (AML) remains the most deadly blood malignancy, with a five-year survival rate of 26% for people over age 20. Children and adolescents fare far better; more than two-thirds of young patients live beyond five years, but they comprise just 4% of all patients. Indeed, AML develops most commonly in individuals between the ages of 65 and 74 among whom the five-year survival rate is less than 5%.
Curative treatment for the disease includes intensive induction chemotherapy followed by consolidation chemotherapy, allogeneic hematopoietic stem-cell transplantation (HCT) or a combination of both, all of which can be difficult to tolerate for the elderly patients typically diagnosed with AML, including most veterans with the disease.
HCT “is a last-resort therapy for acute myeloid leukemia that has a poor prognosis,” noted Anant Madabhushi, PhD, of the Louis Stokes VAMC in Cleveland, and colleagues in a study in JCO Clinical Cancer Informatics. Up to 60% of AML patients achieve complete remission, but then relapse in the absence of consolidation therapy. HCT provides patients a replacement hematopoietic system following immunosuppressive therapy and offers the best chance for a cure.1
HCT is a last-ditch effort, though, because it is associated with high rates of morbidity and mortality as a result of immunosuppression, graft-vs-host disease, infection, organ complications and secondary cancers. Further, “when patients relapse, the prognosis is especially poor, more so in the setting of early relapse where the patient cannot endure further intensive chemotherapy,” the authors said. In addition, salvage therapies including lymphocyte infusions and second HCTs help very few patients who relapse after first transplantation.
Consequently, Madabhushi and colleagues sought to develop a machine learning model that enabled them to predict relapse post-HCT so that aggressive treatments are delivered only to those most likely to need them. “The low response rate, poor improvement under salvage therapy, and substantial side effects of these treatments make it vital to direct them only to patients at high risk of AML relapse,” the team noted.
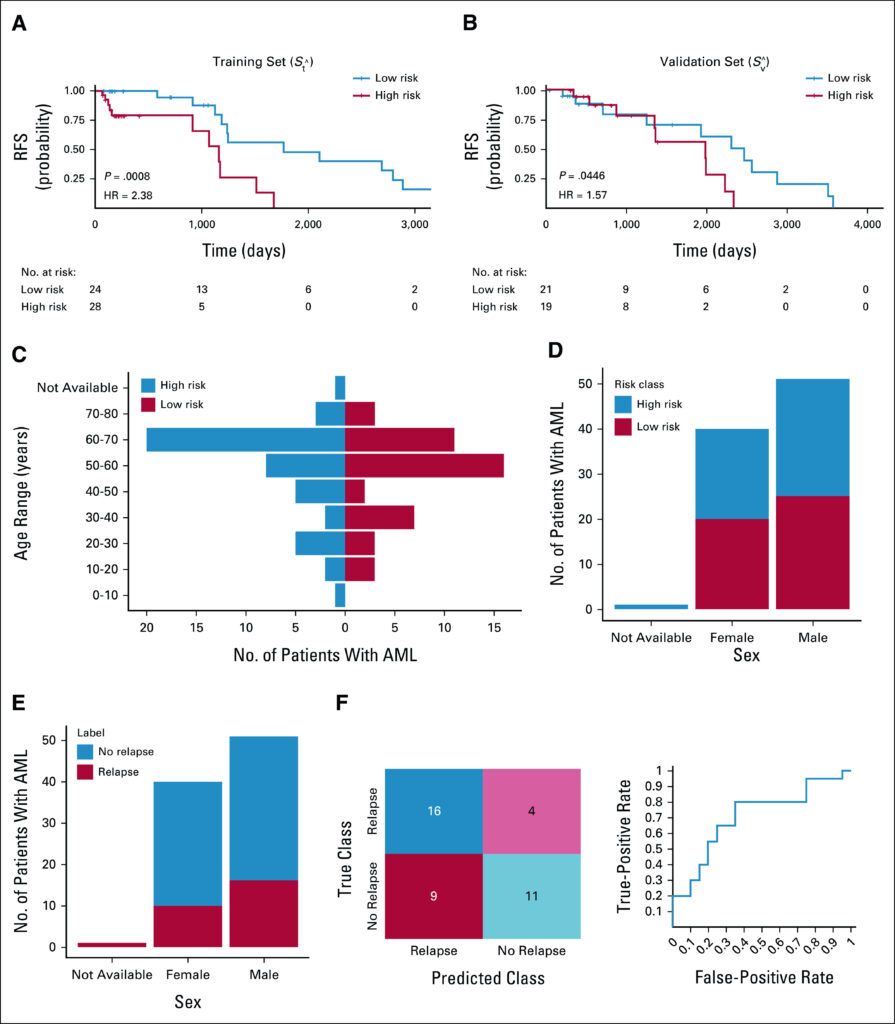
Click to Enlarge: The Kaplan-Meier curves of the high-risk (red) and low-risk groups (blue) in (A) Stˆ (training set; HR = 2.38, 95% CI, 1.43 to 3:95; P = .0008) and (B) Svˆ (validation set; HR = 1.58; 95% CI, 1.01 to 2.4; P = .04); (C) distribution of high-risk and low-risk patients in different age ranges, with (D) and (E) showing the sex distribution in different groups; and (F) the LDA classification results via both a confusion matrix and the ROC curve. AML, acute myeloid leukemia; HR, hazard ratio; LDA, linear discriminant analysis; ROC, receiver operating characteristic curve.
Currently, physicians review aspirates from bone marrow biopsy to determine the bone marrow blast percentage and examine cell morphology. “In this process, a pathologist counts approximately 200-300 cells from randomly chosen regions of a bone marrow aspirate specimen, and if 5% or more of the cells are blasts, the patient is considered to have relapsed,” the authors explained. Human fallibility and the small percentage of cells examined make this process less accurate and predictable than desired, two factors that the research team thought could be addressed by a computational approach that assessed cell features associated with relapse or relapse-free survival (RFS) post-transplant.
“Blast count is a useful predictor of relapse, but clearly insufficient. The findings in our study clearly reveal that quantifying the density of blasts alone is insufficient and that the specific features of shape, texture and spatial architecture of the blasts provide even more prognostic information compared to simply quantifying blast density,” Madabhushi told U.S. Medicine.
“The patients that tend to relapse tend to have the higher texture in turn reflective of higher degree of heterogeneity in turn suggesting more breakdown of morphology and microarchitecture compared to patients with better outcome/prognosis that have the lower texture (smoother morphology),” Madabhushi explained.
Using post-HCT images from 92 patients with AML or its common precursor, myelodysplastic syndrome (MDS), the team created a training set of 52 images and a validation set of 40 images. They then developed a deep learning-based model to segment myeloblasts and extracted 214 texture and shape descriptors from the images of the segmented myeloblasts. Lastly, they established a risk score based on texture features of myeloblast chromatin patterns.
The risk scores generated by the model were associated with relapse-free survival in the training set (hazard ratio = 2.38; 95% CI, 1.4 to 3.95; P = .0008) and the validation set (hazard ratio = 1.57; 95% CI, 1.01 to 2.45; P = .044), indicating that the morphological characteristics and textures were indicative of lower relapse risk. On the flip side, the model also predicted which patients would experience relapse with an area under the receiver operating characteristic curve of 0.71 within the validation set.
“These tools could serve as a companion diagnostic tool to aid in identifying which AML patients are likely to relapse on account of the bone marrow transplant,” said Madabhushi.
The team took an unusually transparent approach to developing the machine-learning model, which they believe provides significant advantages for clinicians.
“The tools we are developing are with an eye towards precision medicine and developing decision support tools to be used by the clinician/oncologist to modulate treatment strategies. While deep learning (DL) strategies might be useful in this regard, the lack of transparency will hinder the ability of the clinician to trust these tools for decision-making,” Madabhushi noted.
“Clinicians are likely to trust and hence use those tools where they understand the underlying representations employed for the classifier. The bigger issue is that the lack of transparency with deep learning also means that it is difficult to debug these approaches when they break down. Another advantage for the transparent approaches over the DL-based approaches is the fact that the transparent approaches require significantly less data for training the predictors.”
- Arabyarmohammadi S, Leo P, Viswanathan VS, Janowczyk A, Corredor G, Fu P, Meyerson H, Metheny L, Madabhushi A. Machine Learning to Predict Risk of Relapse Using Cytologic Image Markers in Patients With Acute Myeloid Leukemia Posthematopoietic Cell Transplantation. JCO Clin Cancer Inform. 2022 May;6:e2100156.